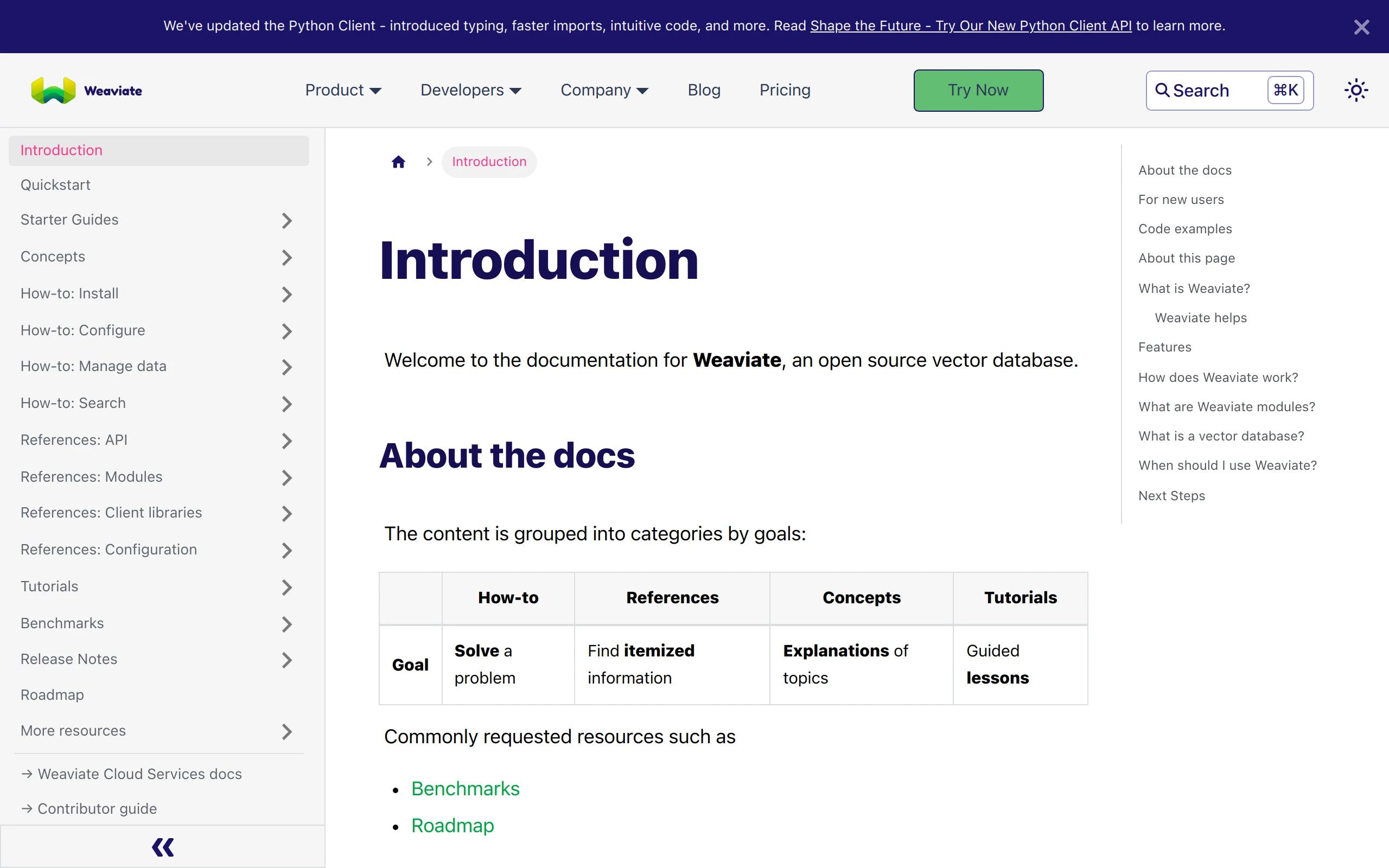
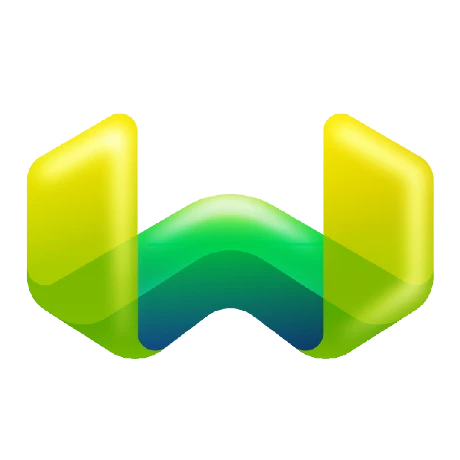
Weaviate
9.6k 638What is Weaviate ?
Weaviate is an open source vector database that stores both objects and vectors, allowing for combining vector search with structured filtering with the fault-tolerance and scalability of a cloud-native database, all accessible through GraphQL, REST, and various language clients.
With Weaviate, you can turn your text, images and more into a searchable vector database using state-of-the-art ML models.
Quick Start with Weaviate
If you just want to get started Try:
-
the quickstart tutorial if you are looking to use Weaviate, or
-
the contributor guide if you are looking to contribute to the project.
And you can find our documentation here.
Weaviate Features
Speed
Weaviate typically performs a 10-NN neighbor search out of millions of objects in single-digit milliseconds. See benchmarks.
Flexibility
You can use Weaviate to conveniently vectorize your data at import time, or alternatively you can upload your own vectors.
These vectorization options are enabled by Weaviate modules. Modules enable use of popular services and model hubs such as OpenAI, Cohere or HuggingFace and much more, including use of local and custom models.
Production-readiness
Weaviate is designed to take you from rapid prototyping all the way to production at scale.
To this end, Weaviate is built with scaling, replication, and security in mind, among others.
Beyond search
Weaviate powers lightning-fast vector searches, but it is capable of much more. Some of its other superpowers include recommendation, summarization, and integrations with neural search frameworks.
What can you build with Weaviate?
For starters, you can build vector databases with text, images, or a combination of both.
You can also build question and answer extraction, summarization and classification systems.
You can see code examples here, and you might find these blog posts useful:
Integrations
Examples and/or documentation of Weaviate integrations (a-z).
-
Auto-GPT (blogpost) – use Weaviate as a memory backend for Auto-GPT
-
DocArray - Use Weaviate as a document store in DocArray.
-
Haystack (blogpost) - Use Weaviate as a document store in Haystack.
-
Hugging Face - Use Hugging Face models with Weaviate.
-
LangChain (blogpost) - Use Weaviate as a memory backend for LangChain.
-
LlamaIndex (blogpost)- Use Weaviate as a memory backend for LlamaIndex.
-
OpenAI - ChatGPT retrieval plugin - Use Weaviate as a memory backend for ChatGPT.
-
OpenAI - use OpenAI embeddings with Weaviate.
Weaviate helps
-
Software Engineers - Who use Weaviate as an ML-first database for your applications.
-
Out-of-the-box modules for: AI-powered searches, Q&A, integrating LLMs with your data, and automatic classification.
-
With full CRUD support like you’re used to from other OSS databases.
-
Cloud-native, distributed, runs well on Kubernetes and scales with your workloads.
-
-
Data Engineers - Who use Weaviate as fast, flexible vector database
-
Use your own ML mode or out-of-the-box ML models, locally or with an inference service.
-
Weaviate takes care of the scalability, so that you don’t have to.
-
-
Data Scientists - Who use Weaviate for a seamless handover of their Machine Learning models to MLOps.
-
Deploy and maintain your ML models in production reliably and efficiently.
-
Easily package any custom trained model you want.
-
Smooth and accelerated handover of your ML models to engineers.
-
Interfaces
You can use Weaviate with any of these clients:
You can also use its GraphQL API to retrieve objects and properties.