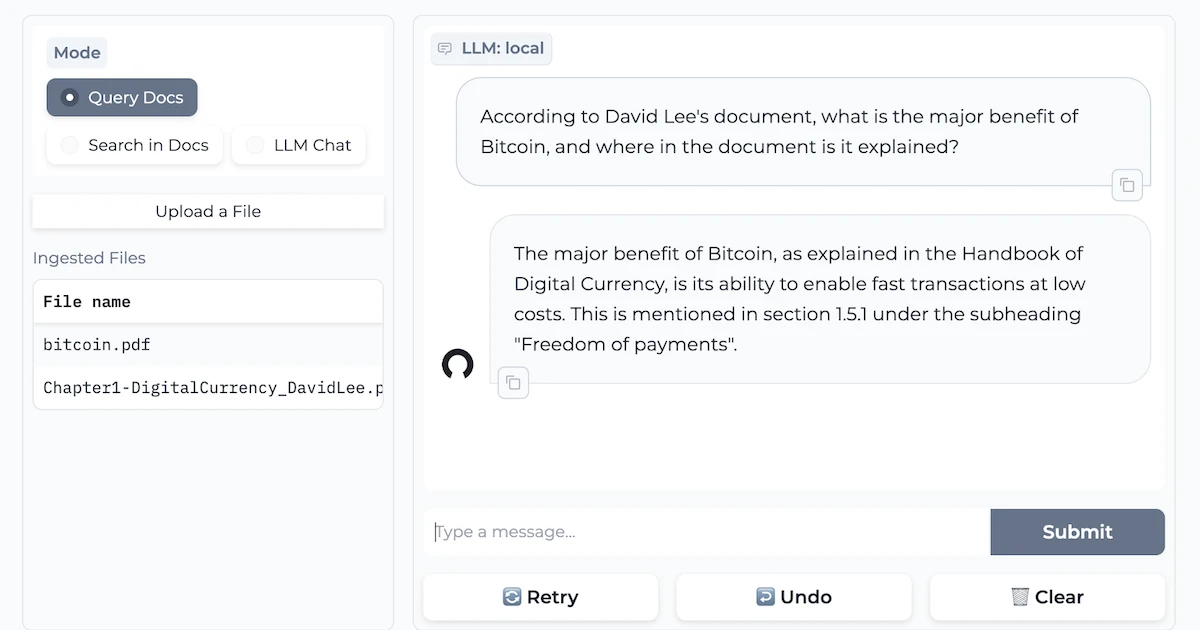
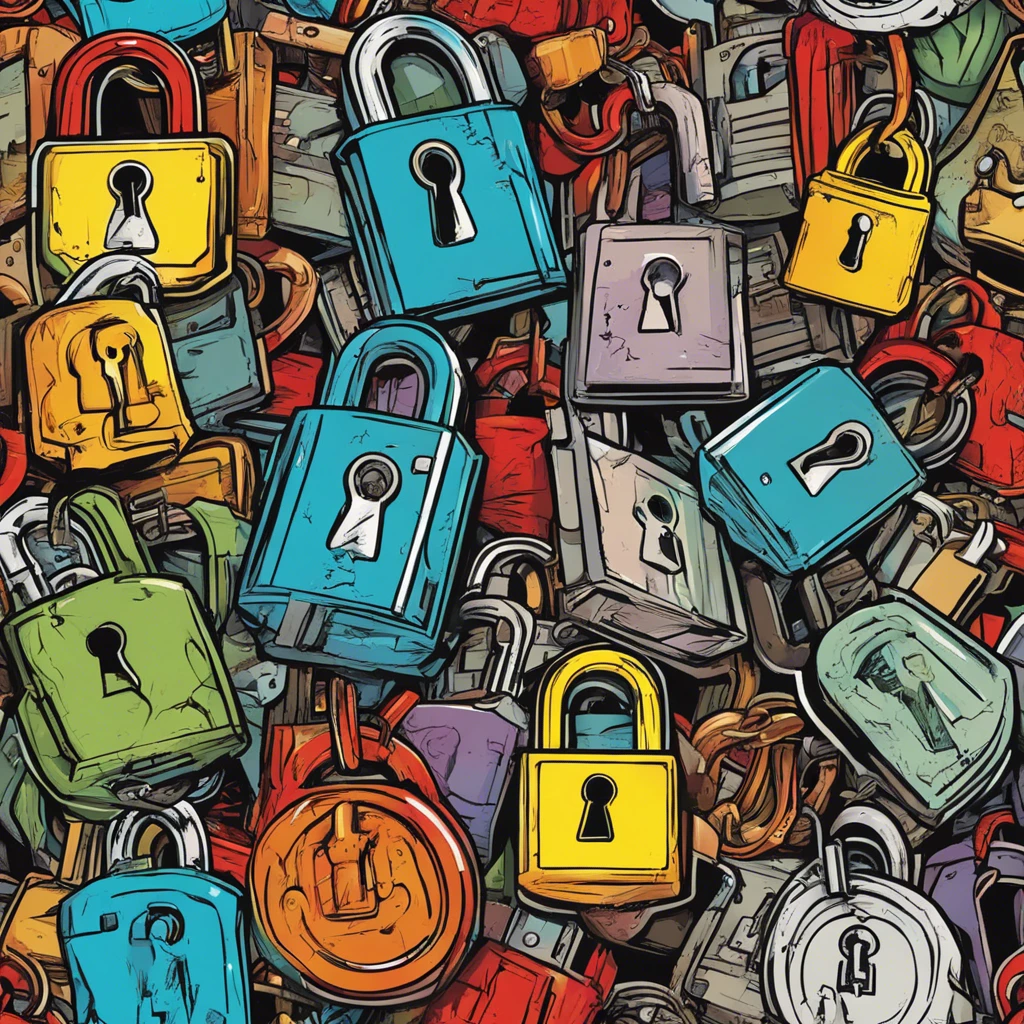
PrivateGPT
51.9k 6.9kWhat is PrivateGPT ?
PrivateGPT is a production-ready AI project that allows you to ask questions about your documents using the power of Large Language Models (LLMs), even in scenarios without an Internet connection. 100% private, no data leaves your execution environment at any point.
PrivateGPT Features
The project provides an API offering all the primitives required to build private, context-aware AI applications.
It follows and extends the OpenAI API standard, and supports both normal and streaming responses.
The API is divided into two logical blocks:
High-level API, which abstracts all the complexity of a RAG (Retrieval Augmented Generation)
pipeline implementation:
- Ingestion of documents: internally managing document parsing,
splitting, metadata extraction, embedding generation and storage.
- Chat & Completions using context from ingested documents:
abstracting the retrieval of context, the prompt engineering and the response generation.
Low-level API, which allows advanced users to implement their own complex pipelines:
-
Embeddings generation: based on a piece of text.
-
Contextual chunks retrieval: given a query, returns the most relevant chunks of text from the ingested documents.
In addition to this, a working Gradio UI
client is provided to test the API, together with a set of useful tools such as bulk model
download script, ingestion script, documents folder watch, etc.
👂 Need help applying PrivateGPT to your specific use case?
and we’ll try to help! We are refining PrivateGPT through your feedback.
🧩 Architecture
Conceptually, PrivateGPT is an API that wraps a RAG pipeline and exposes its
primitives.
-
The API is built using FastAPI and follows
-
The RAG pipeline is based on LlamaIndex.
The design of PrivateGPT allows to easily extend and adapt both the API and the
RAG implementation. Some key architectural decisions are:
-
Dependency Injection, decoupling the different components and layers.
-
Usage of LlamaIndex abstractions such as
LLM
,BaseEmbedding
orVectorStore
,making it immediate to change the actual implementations of those abstractions.
-
Simplicity, adding as few layers and new abstractions as possible.
-
Ready to use, providing a full implementation of the API and RAG
pipeline.
Main building blocks:
- APIs are defined in
private_gpt:server:<api>
. Each package contains an
<api>_router.py
(FastAPI layer) and an <api>_service.py
(the
service implementation). Each Service uses LlamaIndex base abstractions instead
of specific implementations,
decoupling the actual implementation from its usage.
- Components are placed in
private_gpt:components:<component>
. Each Component is in charge of providing
actual implementations to the base abstractions used in the Services - for example
LLMComponent
is in charge of providing an actual implementation of an LLM
(for example LlamaCPP
or OpenAI
).
Install PrivateGPT
Base requirements to run PrivateGPT
Git clone PrivateGPT repository, and navigate to it:
git clone https://github.com/imartinez/privateGPT
cd privateGPT
Install Python 3.11 (if you do not have it already). Ideally through a python version manager like pyenv. Earlier python versions are not supported.
Install make for scripts:
- MacOS: (Using homebrew): ```brew install make
- Windows: (Using chocolatey) ```choco install make```
**Install the dependencies:**
```sh
poetry install --with ui
Verify everything is working by running `make run`
(or poetry run python -m private_gpt) and navigate to http://localhost:8001 You should see a Gradio UI configured with a mock LLM that will echo back the input. Below we’ll see how to configure a real LLM.
For Local LLM requirements check PrivateGPT Docs