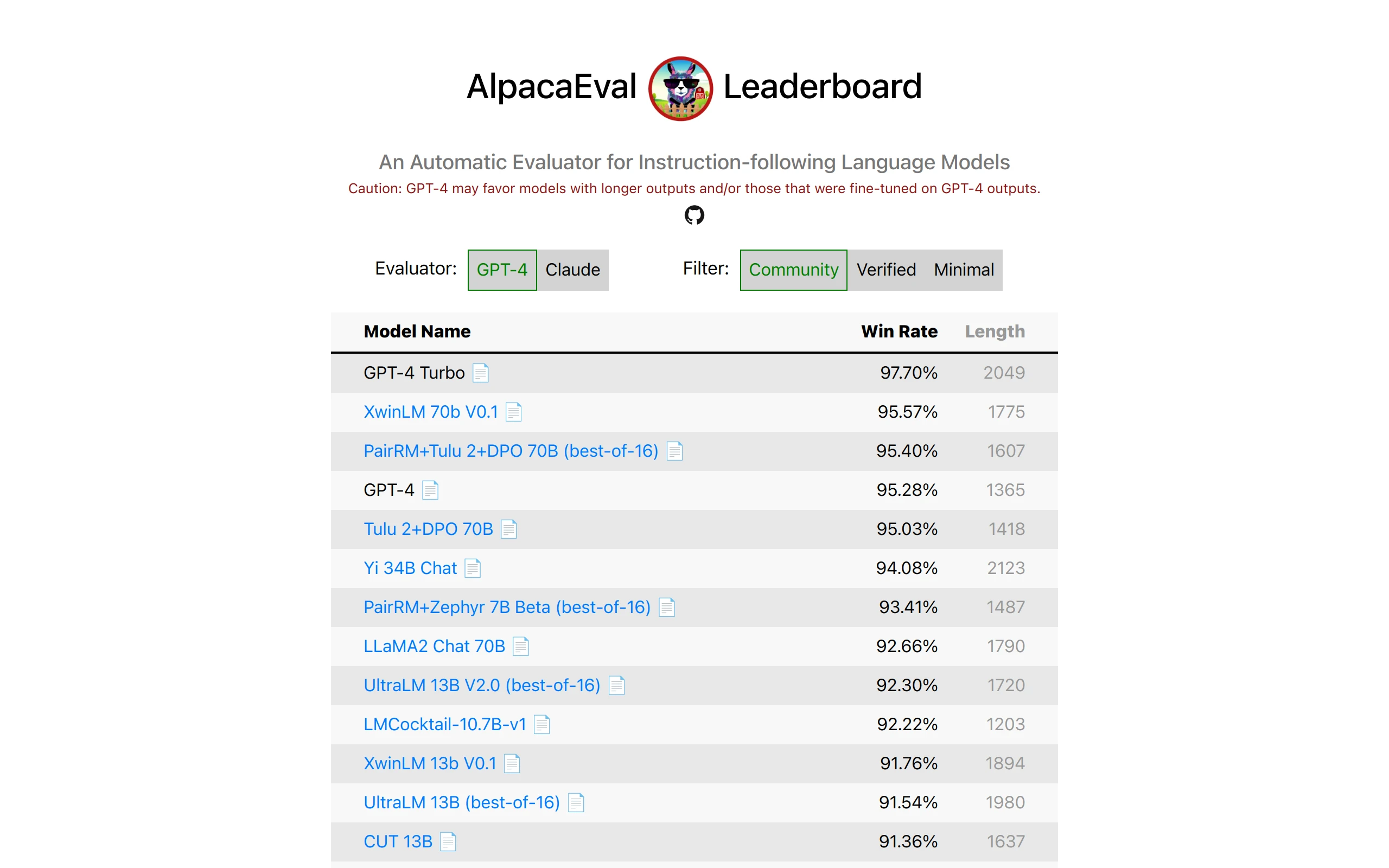
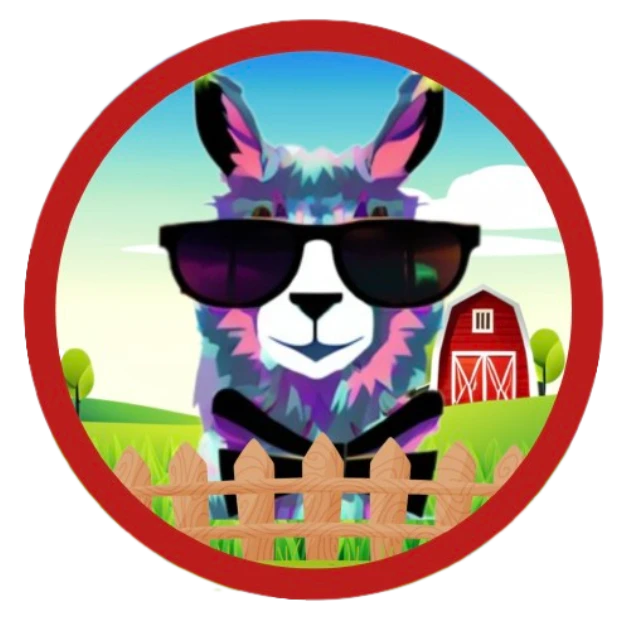
AlpacaEval
1.1k 164What is AlpacaEval ?
AlpacaEval an LLM-based automatic evaluation that is fast, cheap, and reliable. It is based on the AlpacaFarm evaluation set, which tests the ability of models to follow general user instructions. These responses are then compared to reference Davinci003 responses by the provided GPT-4 or Claude or ChatGPT based auto-annotators, which results in the win rates presented above. AlpacaEval displays a high agreement rate with ground truth human annotations, and leaderboard rankings on AlpacaEval are very correlated with leaderboard rankings based on human annotators. Please see our documentation for more details on our analysis. Evaluation of instruction-following models (e.g., ChatGPT) typically requires human interactions. This is time-consuming, expensive, and hard to replicate. AlpacaEval in an LLM-based automatic evaluation that is fast, cheap, replicable, and validated against 20K human annotations. It is particularly useful for model development.
AlpacaEval Features
AlpacaEval provides the following:
- Leaderboard: a leaderboard of common models on the AlpacaEval evaluation set. Caution: Automatic evaluator (e.g. GPT4) may be biased towards models that generate longer outputs and/or that were fine-tuned on the model underlying the evaluator (e.g. GPT4).
- Automatic evaluator: an automatic evaluator that has high agreement with humans (validated on 20K annotations). We evaluate a model by measuring the fraction of times an powerful LLM (e.g. GPT 4 or Claude or ChatGPT) prefers the outputs from that model over outputs from a reference model. Our evaluators enable caching and output randomization by default.
- Toolkit for building automatic evaluators: a simple interface for building advanced automatic evaluators (e.g. with caching, batching, or multi-annotators) and analyzing them (quality, price, speed, statistical power, bias, variance etc).
- Human evaluation data: 20K human preferences between a given and reference model on the AlpacaFarm evaluation set. 2.5K of these are cross-annotations (4 humans annotating the same 650 examples).
- AlpacaEval dataset: a simplification of AlpacaFarm’s evaluation set, where “instructions” and ” inputs” are merged into one field, and reference outputs are longer. Details here. When to use AlpacaEval? Our automatic evaluator is a quick and cheap proxy for human evaluation of simple instruction-following tasks. It is useful if you have to run many evaluations quickly, e.g., during model development. When not to use AlpacaEval? As any other automatic evaluator, AlpacaEval should not replace human evaluation in high-stake decision-making, e.g., to decide on model release. In particular, AlpacaEval is limited by the fact that (1) the instructions in the eval set might not be representative of advanced usage of LLMs; (2) automatic evaluators may have biases such as favoring style over factuality of the answer; and (3) AlpacaEval does not measure the risks that a model could cause. Details in limitations.
Quick Start
To install the stable release, run
pip install alpaca-eval
To install the nightly version, run
pip install git+https://github.com/tatsu-lab/alpaca_eval
Then you can use it as follows:
export OPENAI_API_KEY=<your_api_key> # for more complex configs, e.g. using Azure or switching clients see client_configs/README.mdalpaca_eval --model_outputs 'example/outputs.json'
This will print the leaderboard to the console, and save both the leaderboard and the annotations to the same directory as the model_outputs
file. Important parameters are the following:
- model_outputs : A path to a json file for the outputs of the model to add to the leaderboard. Each dictionary
should
contain the keys
instruction
andoutput
. - annotators_config: This is the annotator to use (e.g.,
alpaca_eval_gpt4
orclaude
orchatgpt_fn
).alpaca_eval_gpt4
( default) has the highest agreement rate with our human annotation data.claude
has a decent agreement and is free for academics.chatgpt_fn
is the worst of the three, but is available to everyone, cheap, and has 2x larger context window (16K tokens). For a comparison of annotators see here. - reference_outputs: The outputs of the reference model. Same format as
model_outputs
. By default, this istext-davinci003
outputs on AlpacaEval dataset. - output_path: Path for saving annotations and leaderboard.
If you don’t have the model outputs, you can
use
evaluate_from_model
and pass a local path or a name of a HuggingFace model, or a model from a standard API (OpenAI, Anthropic, Cohere). Other commands:
SYNOPSIS alpaca_eval COMMANDCOMMANDS COMMAND is one of the following: evaluate Evaluate a model based on its outputs. This is the default entrypoint if no command is specified. evaluate_from_model Evaluate a model from HuggingFace or an API provider. This is a wrapper around `evaluate` which includes generating from a desired model. make_leaderboard Precompute and save an entire leaderboard for a given dataset / evaluator / set of models generations. analyze_evaluators Analyze an evaluator (agreement with human, speed, price,...).
For more information about each function use alpaca_eval <command> -- --help
.
Leaderboards and how to interpret them
Models
Our leaderboards are computed on the AlpacaEval dataset.
We precomputed the leaderboard for important models using alpaca_eval_gpt4
(best quality), claude
(free for
academics, and high quality), and chatgpt_fn
(cheap and available for everyone). Our full leaderboards can be found
at on this page, but
we give minimal leaderboards below.
Later we also show how to add your model to the
leaderboard and how to make
a new leaderboard for your evaluator/dataset.
See here for the configs of all
models that are available out of the box.
** alpaca_eval_gpt4
minimal leaderboard**:
Win Rate | Std Error | |
---|---|---|
gpt4 | 95.3 | 0.7 |
claude | 88.4 | 1.1 |
chatgpt | 86.1 | 1.2 |
wizardlm-13b | 75.3 | 1.5 |
guanaco-65b | 71.8 | 1.6 |
vicuna-13b | 70.4 | 1.6 |
oasst-rlhf-llama-33b | 66.5 | 1.7 |
text_davinci_003 | 50.0 | 0.0 |
falcon-40b-instruct | 45.7 | 1.8 |
alpaca-farm-ppo-human | 41.2 | 1.7 |
alpaca-7b | 26.5 | 1.5 |
text_davinci_001 | 15.2 | 1.2 |
How exactly are those metrics computed?
Win Rate: the win rate measures the fraction of time the model’s output is preferred over text-davinci-003 outputs (
i.e. the reference).
More specifically, to compute the win rate we collect pairs of outputs of the desired model on every instruction from
the
ApacaEval dataset.
We then pair each output with the output of our reference model ( text-davinci-003
) on the same instruction.
We then ask our automatic evaluator which output they prefer.
See here
and here for the exact
prompts and configs for GPT4 and Claude, in particular we randomize the order of
outputs to avoid position bias.
We then average the preferences over all instructions in the dataset to get the win rate of the model over
text-davinci-003.
If both outputs are exactly the same we use a half preference for both models.
Standard error: this is the standard error (normalized by N-1) of the win rate, i.e., the preferences averaged over
the different instructions.
Details about alpaca_eval_gpt4
Our alpaca_eval_gpt4
(
see configs)
annotator averages over preferences, where preferences are obtained as follows:
- it takes in an instruction and a pair of outputs (from the desired model and the reference model)
- if a preference was this triple was already computed, it returns it (i.e. it uses caching)
- it randomizes the order of the outputs to avoid position bias
- it formats the instruction and outputs into the following zero-shot prompt, which asks to order the outputs in order of preference
- it completes the prompt using GPT4 with
temperature=0
- it parses the preference from the completions and returns it The annotator is a mix between (and was highly influenced by) AlpacaFarm and Aviary evaluators. In particular, we use the same code as for AlpacaFarm (caching/randomization/hyperparameters) but use a ranking prompt similar to that of Aviary. We make changes to Aviary’s prompt to decrease the bias for longer outputs. Details in Related work.
claude minimal leaderboard
Win Rate | Std Error | |
---|---|---|
gpt4 | 77.0 | 1.5 |
claude | 75.8 | 1.5 |
chatgpt | 67.7 | 1.6 |
wizardlm-13b | 66.1 | 1.7 |
vicuna-13b | 63.2 | 1.7 |
guanaco-65b | 62.6 | 1.7 |
oasst-rlhf-llama-33b | 57.3 | 1.7 |
text_davinci_003 | 50.0 | 0.0 |
falcon-40b-instruct | 46.7 | 1.8 |
alpaca-farm-ppo-human | 46.5 | 1.8 |
alpaca-7b | 32.3 | 1.6 |
text_davinci_001 | 21.5 | 1.4 |
chatgpt minimal leaderboard
Win Rate | Std Err. | |
---|---|---|
gpt4 | 73.8 | 1.5 |
claude | 70.4 | 1.6 |
chatgpt | 66.1 | 1.7 |
wizardlm-13b | 65.2 | 1.7 |
vicuna-13b | 64.1 | 1.7 |
guanaco-65b | 62.4 | 1.7 |
oasst-rlhf-llama-33b | 62.0 | 1.7 |
alpaca-farm-ppo-human | 60.2 | 1.7 |
falcon-40b-instruct | 56.5 | 1.7 |
text_davinci_003 | 50.0 | 0.0 |
alpaca-7b | 45.2 | 1.7 |
text_davinci_001 | 28.1 | 1.6 |
Evaluators
We evaluate different automatic annotators on the AlpacaEval set by comparing to
2.5K human annotations
we collected (~650 instructions each with 4 human annotations).
Below we show metrics for our suggested evaluator ( alpaca_eval_gpt4
), for prior
automatic
evaluators ( alpaca_farm_greedy_gpt4
, aviary_gpt4
, lmsys_gpt4
),
for humans ( humans
), and for different base models with essentially the same
prompt ( gpt4
, claude
, text_davinci_003
, chatgpt_fn
, guanaco_33b
, chatgpt
).
See here for the configs of all
evaluators that are available out of the box and their associated metrics.
Human agreement [%] | Price [$/1000 examples] | Time [seconds/1000 examples] | Bias | Variance | Proba. prefer longer | |
---|---|---|---|---|---|---|
alpaca_eval_gpt4_fn | 71.0 | 14.5 | 5046 | 27.6 | 11.1 | 0.75 |
alpaca_eval_gpt4 | 69.2 | 13.6 | 1455 | 28.4 | 14.6 | 0.68 |
aviary_gpt4 | 69.1 | 12.8 | 1869 | 29.5 | 13.1 | 0.70 |
gpt4 | 66.9 | 12.5 | 1037 | 31.5 | 14.6 | 0.65 |
alpaca_farm_greedy_gpt4 | 66.4 | 15.3 | 878 | 30.2 | 19.3 | 0.60 |
humans | 65.7 | 300.0 | 36800 | 0.0 | 34.3 | 0.64 |
claude | 65.5 | 11.1 | 173 | 31.9 | 18.0 | 0.62 |
text_davinci_003 | 64.1 | 8.7 | 121 | 33.8 | 22.7 | 0.70 |
lmsys_gpt4 | 63.2 | 13.9 | 17982 | 34.7 | 16.1 | 0.74 |
chatgpt_fn | 60.0 | 1.0 | 530 | 36.9 | 27.7 | 0.62 |
chatgpt | 57.2 | 0.8 | 285 | 39.4 | 34.1 | 0.59 |
How exactly are those metrics computed?
We now explain in words how we compute the metrics in the table
above. The code is here.
Human agreement [%]: this measures the agreement between the current annotator and the majority preferences of
humans on
our
~650 annotations from
our cross-annotation set,
which contains 4 human annotations per example.
To estimate the agreement between a single human ( humans
row in the table above) and the majority of humans, we take
one of the 4 annotations and compute the accuracy that it has when predicting the mode of the other 3 annotations.
We then average this accuracy over all 4 annotations and over the 650 instructions to get the human agreement, i.e., we
compute the expected (over humans and samples)
leave-one-out agreement.
If the mode is not unique, we take one of the modes at random.
We perform exactly the same computation for the automatic annotators, so that the final numbers are comparable.
Price [$/1000 examples]: this is the average price of every 1000 annotations.
For humans, it is the price that we paid Mechanical Turkers to collect those
annotations ($18/hour).
If the price depends on the machine used to compute the annotations (e.g. Guanaco) we leave it empty.
Time [seconds/1000 examples]: this is the average time it takes to compute 1000 annotations.
For humans, it is the estimated median time that each Mechanical Turker took to annotate 1000 examples.
For automatic annotators, it is the average time that it took us when running the annotations. Note that this can depend
on API limits that are different for different users and the number of requests that the clusters are
processing.
Bias: agreement between the most likely human label and the most likely automatic one.
For automatic annotators we estimate it by sampling 4 different annotations for each example.
The randomness here comes from the order of the outputs in the prompt, sampling from the LLM, and if applicable the
order of the instruction in the batch and the choice of annotator in the pool.
We then take the mode of the 4 annotations and compute the accuracy of the mode when predicting the mode of the 4 human
annotations.
Note that this is likely an overestimate on the real bias that we would get if we had an “infinite” number of
cross-annotations.
A low bias means that the annotator has in expectation the same preferences as humans.
For the case of humans, the bias is zero by definition.
Note that this is related to but not the standard statistical bias, because we take the mode instead of average over
annotations and we consider 0-1 loss instead of squared loss.
Variance: expected agreement a single automatic preference and the most likely one.
We estimate it the same way as we estimated “human agreement” for humans, i.e., we take the expected leave one out error
when predicting the mode of the 3 annotations using the 4th annotation.
A low variance means that the annotator is consistent with its preference, i.e., if you sample from it with different
seeds it will give the same result.
As with the bias, this is not exactly the standard statistical variance, because we take the mode instead of average
over annotations and we
consider 0-1 loss instead of squared loss.
Note that the “human agreement” is tightly related to the bias and variance. In particular, the variance
measures the error due to the fact that we only use a single annotation while the bias aims to measure the irreducible
error
for the current annotator.
Proba. prefer longer: this is the probability that the annotator prefers the longer output when one of the two
outputs is significantly longer than the other (more than 30 characters difference).
In the full table we
also provide the following metrics:
Proba. prefer lists: this is the probability that the annotator prefers the output that contains a list/bullet
points when one output does but not the other.
Proba. prefer 1: this is the probability that the annotator prefers the first of the pair of outputs. All our
proposed annotators randomize over outputs in the prompt, so this should be 0.5. Prior annotators, such as lmsys
and aviary
, do not.
# parsed: this is the number of examples that the annotator was able to parse.
Note that if the variance and bias is empty, it means that we only performed one single annotation for each 648 example
due to resource (time and price) constraints. This explains why the #parsed is 648, otherwise it should be 2592.
Tips for choosing evaluators
Overall we recommend using annotators_config=alpaca_eval_gpt4
if you want the highest agreement with humans,
annotators_config=claude
if you have academic (free) access to Claude and have a low budget, and
annotators_config=chatgpt_fn
if you don’t have access to the other two models.
When choosing an annotator we recommend you to consider the following (the first three are obvious):
"Human agreement [%]"
"Price [$/1000 examples]"
"Time [seconds/1000 examples]"
"Proba. prefer longer"
approx. < 0.7. Indeed, we found see that the majority of preference of human annotators have strong bias for longer answers (as shown by the high performance=62.2 of the"longest"
evaluator that always prefers the longest output). This suggests that it might more of a bias with the human annotators. In order to avoid having leaderboards with strong biases for length, we suggest using automatic annotators with less than 0.7 “Proba. prefer longer”."Variance"
approx. < 0.2. We believe that a good evaluator should have as little variance as possible so that results are mostly reproducible. Note that variance can be desirable in the case where we are simulating humans as shown in AlpacaFarm. We filtered the annotators that do not satisfy those requirements in the table above (besides humans / ChatGPT / 003 / lmsys for reference purposes). For all results see here. In general, we foundalpaca_eval_gpt4
to be a good trade-off between quality / price / time / variance / length bias. The above metrics are computed with respect to annotations from crowd-workers. Although useful, those annotations are not perfect, e.g., crowd-workers often favor style over factuality. We thus recommend users to validate automatic evaluators on their own instructions and human annotations. Details in limitations.